Hi, my name is Alex Richter (she/her). I am a recent graduate from Reed College and post bac researcher with Anna Ritz. This is my second summer doing research with Anna Ritz. The project of this summer dealt with letters of recommendation and the role of gendered words/phrases.
The Problem
There are certain words and ways of describing people that appear in letters of recommendation that are gendered. These issues are implicit and occur regardless of the race, gender, or ethnicity of the writer. This issues leads to a disparity of women in specific fields due to the importance of letters of recommendation in graduate school acceptances and hiring.
A very easy example is that women are more likely to be praised in letters of recommendation for their communication or being nice. Men on the other hand are likely to be praised on their ability to do a task or a skill that is relevant to the position. It should also be noted that most of the research in this topic deals with a gender binary of just men and women. Our work tries to avoid the binary by focusing on pronouns, but it should be noted that in discussing this topic phrases such as “Male vs Female” or “Man vs Woman” are common place.
Why does it matter?
Letters of recommendation are important as they allow a better insight into a persons’ character. It is an important factor in hiring a candidate or accepting a candidate into any program. It shows more than just the accomplishments but speaks to who the individual is and how they are perceived by someone who knows them.
This work matters especially at Reed because of the emphasis we put on letters of recommendation. Notoriously, Reed does not give out grades unless requested and while transcripts with letter grades exist the letter grade concept is de-emphasized here. Reed focuses on quality and understanding as opposed to emphasizing grades. As stated in the letter that accompanies all transcripts, “Since its founding, Reed College has adhered to a distinctive educational philosophy, a critical component of which is the evaluation and grading of student academic work.”
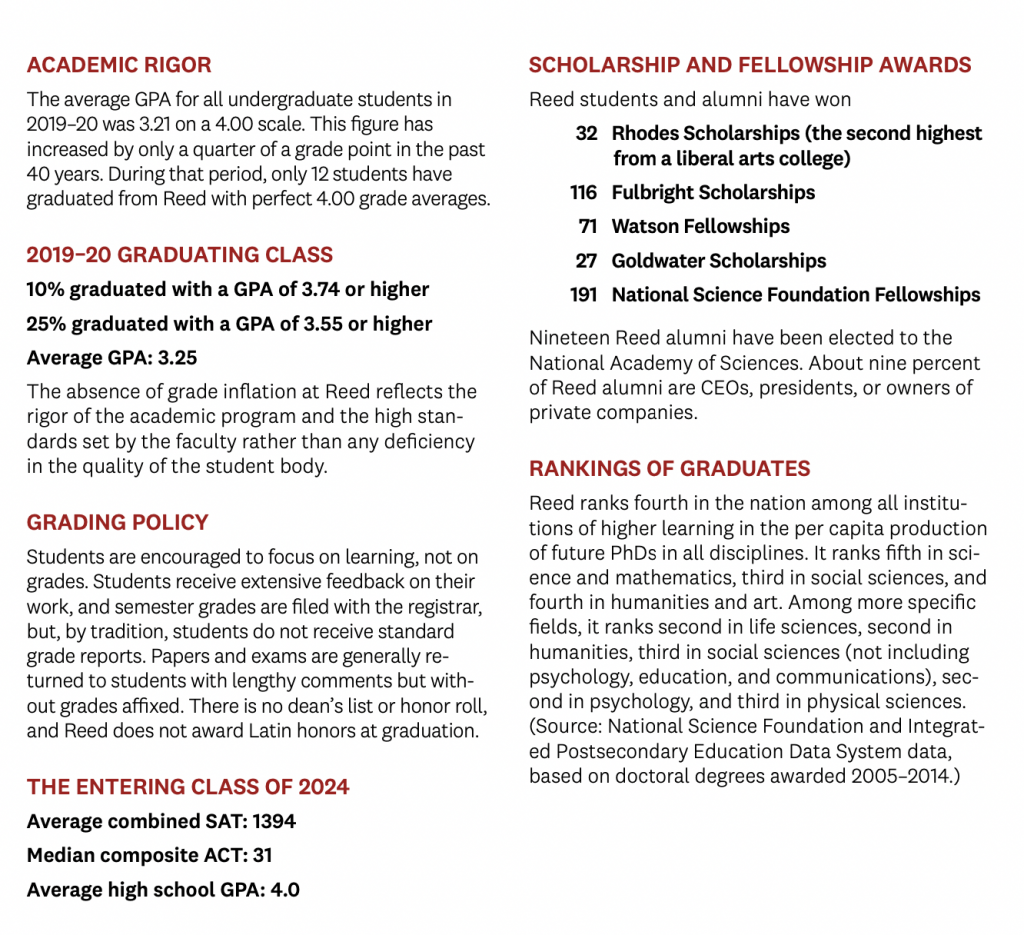
The Pre-Existing Work
In 2018, there was a project done by a graduate student named Mollie Marr and other collaborators. The repository for this project can be found here. This work entailed writing detectors that could be run in order to see if specific words were present in letters of recommendation. There were many detectors that were written by multiple developers. Each detector had been extensively researched and was backed by various publications.
The preexisting detectors that were implemented or suggested:
– Female Detector / Male Detector: Identifies gendered female or male terms respectively.
– Gendered Words Detector: Identifies whether the gender of the candidate is being brought to attention unnecessarily.
– Personal Life Detector: Letters of recommendation for women are more likely to mention facts about their personal life.
– Publication Detector: Letters of recommendation for women are less likely to mention publications and projects.
– Effort (vs Accomplishment) Detector: This one is from a very impactful paper in this topic by Trix and Psenka. Women are more likely to be described using effort words while men are more likely to be described as accomplished or having an innate ability to do the task required.
– Superlative Detector: Letters for women are less likely to contain superlatives. If they do, they usually describe women in the context of emotional terms.
– Conditional Superlative Detector: This deals with superlatives that are “hedged” by restricting the population to only women.
The summer work for this project is dealing primarily with implementing more detectors and making this into an easily accessible resource for people. Future work would deal with creating a google docs or Microsoft word plug in or website. A major issue in this work has been dealing with the lack of a cohesive data set of letters of recommendation. This is due to the difficulty in anonymizing letters of recommendation.
Below is a list of citations that was included in the genderbias repository for any desired future inquiry into this problem.
Publications, Projects, and Research
Trix, F. & Psenka, C., “Exploring the color of glass: Letters of recommendation for female and male medical faculty,” Discourse & Society 14(2003): 191-220.
Superlatives
Dutt, K., Pfaff, D. L., Bernstein, A. F., Dillard, J. S., & Block, C. J. (2016). Gender differences in recommendation letters for postdoctoral fellowships in geoscience. Nature Geoscience, 9(11), 805.
Schmader, T., Whitehead, J., & Wysocki, V. H. (2007). A linguistic comparison of letters of recommendation for male and female chemistry and biochemistry job applicants. Sex Roles, 57(7-8), 509-514.
Nouns
Trix, F. & Psenka, C., “Exploring the color of glass: Letters of recommendation for female and male medical faculty,” Discourse & Society 14(2003): 191-220.
Minimal Assurance
Isaac, C., Chertoff, J., Lee, B., & Carnes, M. (2011). Do students’ and authors’ genders affect evaluations? A linguistic analysis of medical student performance evaluations. Academic Medicine, 86(1), 59.
Trix, F. & Psenka, C., “Exploring the color of glass: Letters of recommendation for female and male medical faculty,” Discourse & Society 14(2003): 191-220.
Effort
Deaux, K. & Emswiller, T., “Explanations of successful performance on sex-linked tasks: What is skill for the male is luck for the female,” Journal of Personality and Social Psychology 29(1974): 80-85
Isaac, C., Chertoff, J., Lee, B., & Carnes, M. (2011). Do students’ and authors’ genders affect evaluations? A linguistic analysis of medical student performance evaluations. Academic Medicine, 86(1), 59.
Steinpreis, R., Anders, K.A., & Ritzke, D., “The impact of gender on the review of the curricula vitae of job applicants and tenure candidates: A national empirical study,” Sex Roles 41(1999): 509-528
Personal Life
Isaac, C., Chertoff, J., Lee, B., & Carnes, M. (2011). Do students’ and authors’ genders affect evaluations? A linguistic analysis of medical student performance evaluations. Academic Medicine, 86(1), 59.
Madera, J. M., Hebl, M. R., & Martin, R. C. (2009). Gender and letters of recommendation for academia: Agentic and communal differences. Journal of Applied Psychology, 94(6), 1591.
Trix, F. & Psenka, C., “Exploring the color of glass: Letters of recommendation for female and male medical faculty,” Discourse & Society 14(2003): 191-220.
Gender Stereotypes
Axelson RD, Solow CM, Ferguson KJ, Cohen MB. Assessing implicit gender bias in Medical Student Performance Evaluations. Eval Health Prof. 2010 Sep;33(3):365-85.
Eagly, A.H.; Karau, S.J., “Role congruity theory of prejudice toward female leaders,” Psychological Review 109, no. 3 (July 2002): 573-597.; Ridgeway, 2002.
Foschi M. Double standards for competence: theory and research. Ann Rev Soc. 2000;26:21–42.
Gaucher, D., Friesen, J., & Kay, A. C. (2011, March 7). Evidence That Gendered Wording in Job Advertisements Exists and Sustains Gender Inequality. Journal of Personality and Social Psychology.
Hirshfield LE. ‘‘She’s not good with crying’’: the effect of gender expectations on graduate students’ assessments of their principal investigators. Gender Educ. 2014;26(6):601–617.
Madera, J. M., Hebl, M. R., & Martin, R. C. (2009). Gender and letters of recommendation for academia: Agentic and communal differences. Journal of Applied Psychology, 94(6), 1591.
Ross DA, Boatright D, Nunez-Smith M, Jordan A, Chekroud A, Moore EZ (2017) Differences in words used to describe racial and gender groups in Medical Student Performance Evaluations. PLoS ONE 12(8): e0181659.
Sprague J, Massoni K. Student evaluations and gendered expectations: what we can’t count can hurt us. Sex Roles. 2005;53(11):779–793.
Steinpreis RE, Anders KA, Ritzke D. The impact of gender on the review of the curricula vitae of job applicants and tenure candidates: a national empirical study. Sex Roles. 1999;41(7):509–528.
Trix, F. & Psenka, C., “Exploring the color of glass: Letters of recommendation for female and male medical faculty,” Discourse & Society 14(2003): 191-220.
Wenneras C, Wold A. Nepotism and sexism in peer review. Nature. 1997;387(6631):341–343.
Raise Doubt
Trix, F. & Psenka, C., “Exploring the color of glass: Letters of recommendation for female and male medical faculty,” Discourse & Society 14(2003): 191-220.
Madera, J. M., Hebl, M. R., Dial, H., Martin, R., & Valian, V. (2019). Raising doubt in letters of recommendation for academia: gender differences and their impact. Journal of Business and Psychology, 34(3), 287-303.
Shorter Length of Letters
Dutt, K., Pfaff, D. L., Bernstein, A. F., Dillard, J. S., & Block, C. J. (2016). Gender differences in recommendation letters for postdoctoral fellowships in geoscience. Nature Geoscience, 9(11), 805.
Trix, F. & Psenka, C., “Exploring the color of glass: Letters of recommendation for female and male medical faculty,” Discourse & Society 14(2003): 191-220.